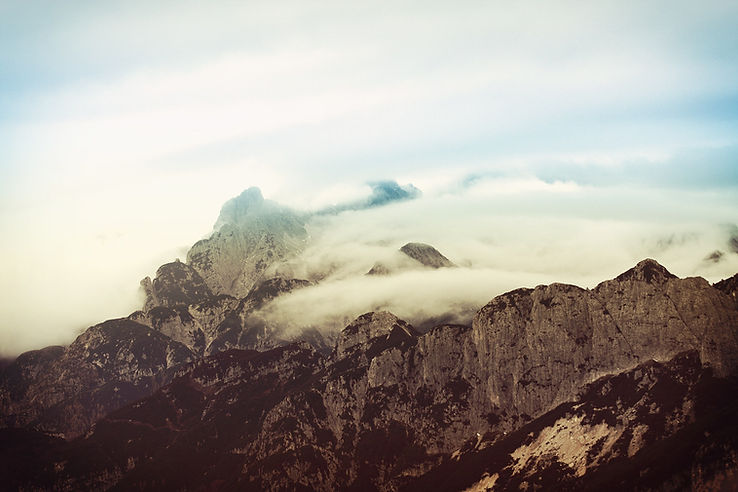
Image Clustering Using Alignable Differences
for Geolocation
(Login: 1, 1, 1)
GEOLOCATION
Geolocation is the task of uncovering the precise geo coordinates of an object - often an image or artifact. It has many applications, ranging from government intelligence to promote national security and journalism efforts to uncover truth, to historical pursuits for museums.
CROWD SOURCING
To increase productivity and spread work across different kinds of talent, many domains are now using crowdsourcing. This is the practice of breaking up work into many different micro tasks, allocating them to a multitude of people, called crowd workers, and then aggregating the results.
OUR GOAL
In the Crowd Intelligence Lab at Virginia Tech, we are interested in finding innovative ways to apply the power of crowdsourcing to the world around us. Geolocation is no exception. As a part of the geolocate team, it was my job to investigate whether or not image clustering had any promise to improve crowd workers performance in geolocation.

THE ALIGNABLE DIFFERENCE
At the start of my research, I conducted some trial studies to investigate if people even had the capacity to recognize image clusters. Participants were shown an image of a place and asked to guess its location, then shown images clustered by country and asked to guess again. The findings were inconclusive - only able to show that the criteria participants used for comparison was not systematically applied to all images, different for each person and often focused in on unhelpful features of the images (ie. using weather or sky color that often changes).
​
From there, I decided to read research papers on how people make comparisons, specifically literary studies of how humans process analogies. Here I found the direction for our project, using cognitive techniques like the alignable difference within our image clusters. This is basically the idea that when two things are more alike, it is actually easier to draw comparisons from them. For example, in the cartoon on the left it is easier to spot the differences between the two frogs rather than the cow and the frog. From this, I realized that we could introduce alignable differences into our image clusters in order to direct crowd workers attention to the similarities and differences that were important for systematic image comparison.
​
OUR TECHNIQUE
For our tool to be applicable, I needed to both develop the alingnable difference's role further and harmonize it with crowdsourcing - namely come up with a micro tasks that could be aggregated in a helpful way. After a lot of brainstorming and more trial studies, I settled on having crowd workers study a mystery image and choose similar images, whose locations would be aggregated into a georeferencing map. Such a map would give clues to the mystery image's relationships to different places, and ultimately its location through studying these relationships and clustering of geo pins.
To help guide crowd workers, we would give image subsets based around alignable differences - to introduce the criteria of comparison. Our hope was that this would help crowd workers make systematic comparisons between images, and ones about features that mattered that had been identified in prior research by the lab. For instance, if a mystery image contained a cow then a crowd worker would be given a set of images of cows, which would help him pay attention to the cows and select alike cow images rather than a lesser attribute like the sky.
THE TASK
For the experiment, I created the interface on the right that showcases a mystery image and 30 images from a image cluster. Each cluster was based on one alignable difference from the mystery image (for example: sari, bicycle, cow) - and had images from a range of distances from the target. From these subsets, 4 images were chosen according to the directions which varied.
Participants were asked to use the think aloud technique as they made comparisons and chose images from each subset . In total we had 20 participants, half for the location direction set, and half for the similarity set - because we were unsure which lean would make better comparisons.
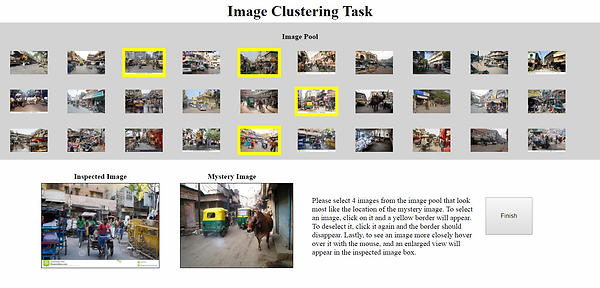

RESULTS
In the end, our hypothesis proved mostly correct. For all subsets (except one) with both direction sets, at least 50% of the locations of picked images were within the first 2 zones - within 100 miles of the target image. Even more impressive, some subsets had as high as 85% within the first 2 zones with 70% of the data within the first zone alone - within 5 miles of the target. In addition, the think aloud technique revealed that participants were using the alignable differences provided to make their selections, as well as some other that were present in the images to make final selections. Across the board, participants highly valued images that featured an urban, commercial crowded district. However, many participants still struggled with making systematic comparisons, or returning to images that they were considering at one time. To investigate improving this method, I am considering employing a tournament comparison style to help. In addition, further research must be done to identify why some alignable differences - like regional clothing - worked worse than others, and what kinds of mystery images would be most effective with this method.
(Charts below: blue - zone 1, red - zone 2, orange - zone 3, green - zone 4, purple - zone 5)





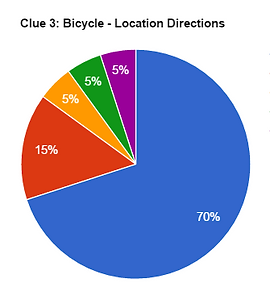
GEOREFERENCING MAP
Zones are marked by the pink circles, the red star marks the mystery image and the pins mark picked image locations:
Zone 1: Regional City
( < 5 mi)
Zone 2: Intersection of Nepal
(< 100 mi)
Zone 3: Neighboring Countries
(< 1000 mi)
Zone 4: Central Asia
(< 2500 mi)
Zone 5: Rest of the World
(> 2500 mi)